AI Offers Support to Clinical Breast Imaging Radiologists
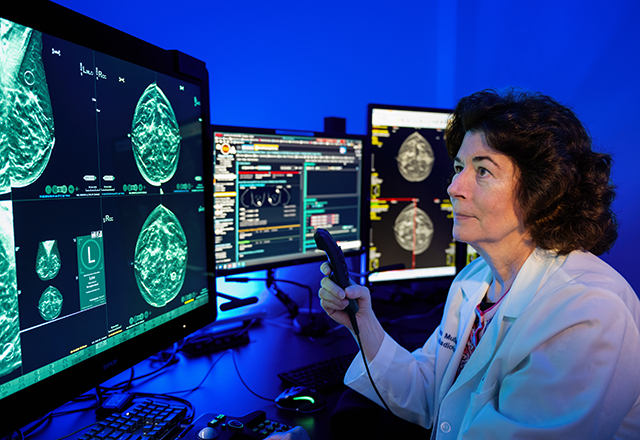
Artificial intelligence is everywhere. The rapidly emerging technology is affecting nearly every field, and radiology is no exception.
In recent years, the Johns Hopkins Department of Radiology and Radiological Science has been exploring AI’s transformational potential through initiatives like the Radiology Artificial Intelligence Development (RAID) Committee. One of RAID’s first initiatives, led by Lisa Mullen, associate professor of radiology and breast imaging fellowship director, is focused on using AI for decision support in mammography interpretation. In March 2024, the committee’s efforts took a giant leap forward as the department’s first AI-assisted detection algorithm for mammography was introduced in the clinical setting.
The FDA-cleared AI software was developed by a third-party vendor. According to Mullen, the department’s vetting process for potential vendors was thorough. Mullen and her team investigated various vendors and their products. They also sent anonymized cases to different vendors to analyze using their software. One vendor’s software performed better, and after a period of preparation, that software was implemented for clinical use.
While the software has only been in use clinically for a few months, Mullen is excited about the potential of the new technology to aid radiologists. AI in the reading room involves using algorithms to analyze large amounts of information. This can assist radiologists by helping detect and characterize lesions, improving diagnostic confidence. The AI algorithm does not use patient data to learn in real time, and no patient information is retained by the software.
Breast imaging radiologists read both 2D and digital breast tomosynthesis (DBT) mammograms (also called 3D), though DBT exams now make up more than 95% of the mammograms performed at Johns Hopkins. During a DBT mammogram, multiple low dose X-ray images are obtained at various angles and then reconstructed into thin (1 millimeter) slices. This means that today’s radiologists have a lot of images to read! The new AI software can help increase radiologists’ efficiency by highlighting areas of concern on the images. The AI algorithm detects possible lesions and gives a probability of malignancy score for each detected finding. The algorithm also produces an overall case score.
The AI software is a decision support tool for radiologists, not a replacement. Right now, Mullen said, AI does not perform at the level of human radiologists. There are currently several limitations to AI-assisted analysis that still require human expertise.
For one, the AI software does not compare the current exam to a patient’s prior mammograms. While AI analyzes each lesion in isolation, our Johns Hopkins radiologists can analyze the images in context. If a lesion has been stable for years, there is less cause for concern than if a new lesion appears or grows significantly. AI is a valuable tool in that it can flag potential issues, saving the radiologist time, but human expertise is still critical.
Another area in which the trained human eye is key is in comparing breasts side to side. Radiologists compare the left and right breasts when reading an exam, examining symmetry and comparing to past exams. Currently, AI software does not compare breasts side to side. Also, the AI software evaluates one image at a time, but the radiologist integrates information from more than one mammographic view to decide about a finding.
While there are some concerns that the software’s implementation may initially result in more callbacks for patients, it should not be a long-term issue. AI adds another layer of findings for radiologists to either recall or dismiss, but any increase in callbacks should be temporary as radiologists adjust to the new technology. As Mullen noted, increased recalls often occur after introduction of new technology, and this issue is not unique to AI.
“I’ve been at Johns Hopkins for more than 20 years, and we’ve been through many different types of technology,” Mullen said, adding, “When I first joined Johns Hopkins, we were using film-screen mammography, then came 2D, then DBT and so on. Every time you transition to a new technology, the callback rate temporarily goes up.”
Over time, the new software should lower the callback rate as AI analysis adds confidence to radiologists’ findings, but this is not an immediate effect.
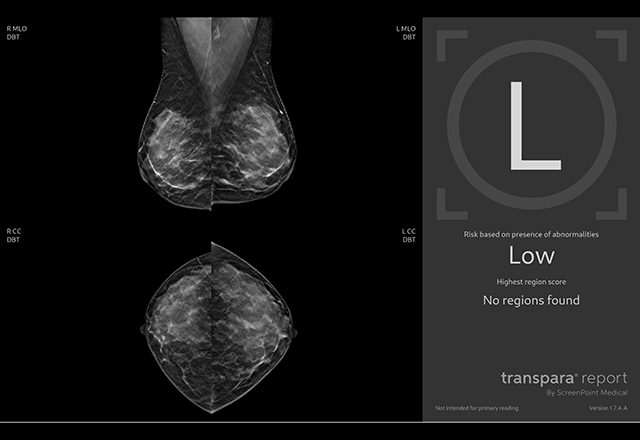
Since the software went live clinically only a few months ago, Mullen said that breast imaging radiologists are still integrating the new tool into their routines.
“We’re in the early stages of AI use,” Mullen said. “We are still figuring out how best to use this product.”
At Johns Hopkins, the new AI-assisted software is applied to every screening and diagnostic mammogram. Patients pay no additional cost for the service.
Mullen is enthusiastic about the possibility of working with the software developer on new research opportunities for the breast imaging division. This could include examining how the AI algorithm affects clinical practice, comparing data from before the software was used and a year after it was implemented. It is still early, but Mullen is looking forward to building scholarship in the field.
She is also optimistic about the future of AI in mammography and medical imaging. Work is already in progress to improve the algorithm to compare to prior mammograms. The software is not a final product. It continues to improve, and will be updated periodically, offering more data for radiologists to hone their readings. The aim is not for the software to read images autonomously but to aid the expert human radiologists in their interpretations.
“This is not a self-driving car,” Mullen said, explaining that fully qualified radiologists read each exam very carefully, and use the output of the AI algorithm as a decision support tool.
With advancements coming every day, Mullen and her team are excited about the potential for AI-assisted analysis to improve breast cancer detection.